We're scaling synthetic reasoning
- Post-training is unlocking new capabilities in foundation models.
- Training on raw human data doesn’t scale.
- Current methods of “alignment” are insufficient;
evaluations are even worse. - Human intent is rich in preferences, collapsed by uniform models.
- AI`s potential hinges on trust, from interpretable data to every layer built upon it.
- Your models should adapt and scale, automatically.
Frontier challenges in AI post-training
Dakota Mahan, Duy Van Phung, Rafael Rafailov, Chase Blagden, Nathan Lile, Louis Castricato, Jan-Philipp Fränken, Chelsea Finn, Alon Albalak
Reinforcement Learning from Human Feedback (RLHF) has greatly improved the performance of modern Large Language Models (LLMs). The RLHF process is resource-intensive and technically challenging, generally requiring a large collection of human preference labels over model-generated outputs. Reinforcement Learning from AI Feedback (RLAIF) addresses this data collection challenge by leveraging synthetic preferences generated by an LLM. However, recent work has shown that synthetic preferences labels may not align well with human preference judgments. To address this, we propose a hybrid approach that unifies RLHF and RLAIF methodologies.
Democratizing AI research is essential, as the future of transformative technologies should not be confined to the corridors of a few profit-driven entities, but open to independent inquiry and understanding for the collective good.
Louis Castricato, Nathan Lile, Suraj Anand, Hailey Schoelkopf, Siddharth Verma, and Stella Biderman
Existing methods for controlling language models, such as RLHF and Constitutional AI, involve determining which LLM behaviors are desirable and training them into a language model. However, in many cases, it is desirable for LLMs to be controllable at inference time, so that they can be used in multiple contexts with diverse needs. We illustrate this with the Pink Elephant Problem: instructing an LLM to avoid discussing a certain entity (a “Pink Elephant”), and instead discuss a preferred entity (“Grey Elephant”).
Supported By
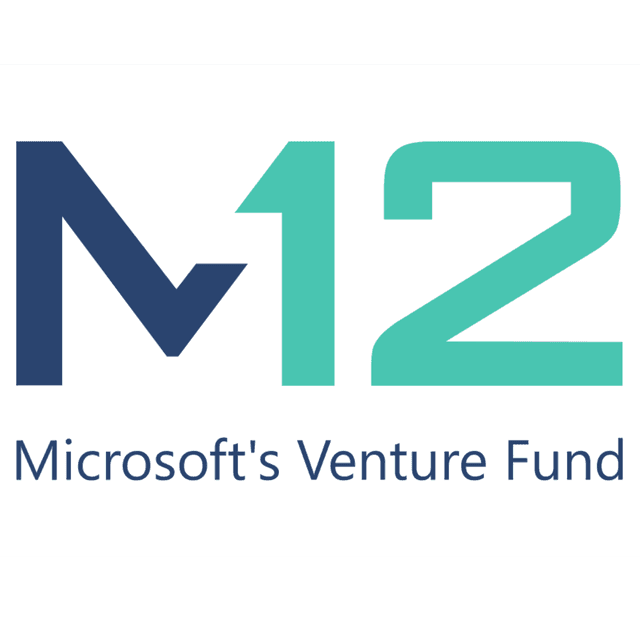
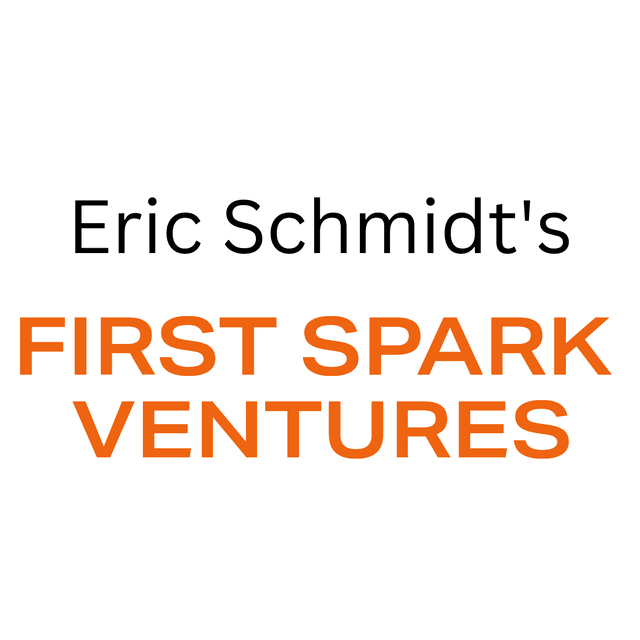